The integration of Natural Language Processing (NLP) into predictive maintenance strategies in mechanical engineering represents a significant advancement in industrial efficiency and operational reliability.
NLP enables the extraction, interpretation, and utilization of textual data, facilitating improved decision-making in maintenance processes. By leveraging NLP, industries can process unstructured maintenance logs, technical manuals, sensor data reports, and operator feedback to enhance equipment reliability and reduce downtime.
One of the most impactful contributions of NLP in predictive maintenance is its ability to analyze maintenance logs and historical reports. Traditional maintenance records often contain vast amounts of textual data, written in varied formats and terminologies. NLP algorithms can parse this data, identify patterns, and extract relevant insights regarding recurring failures, component degradation, and effective past interventions. This allows maintenance teams to anticipate potential failures before they occur, optimizing scheduling and resource allocation.
Another key aspect of NLP application in predictive maintenance lies in its ability to process real-time textual feedback from operators and technicians. Field reports often contain valuable observations about machinery performance, anomalies, and environmental conditions affecting mechanical components. NLP models can classify and quantify these reports, enabling predictive models to factor in human expertise alongside sensor-based data. This enhances the accuracy of maintenance predictions, leading to proactive interventions rather than reactive repairs.
Technical documentation and manuals serve as crucial references for maintenance activities, but navigating these large volumes of text efficiently can be challenging. NLP-driven search and summarization tools enable engineers to retrieve precise information quickly, reducing the time required for diagnostics and troubleshooting. This capability enhances workforce productivity and ensures that maintenance actions align with best practices specified by equipment manufacturers.
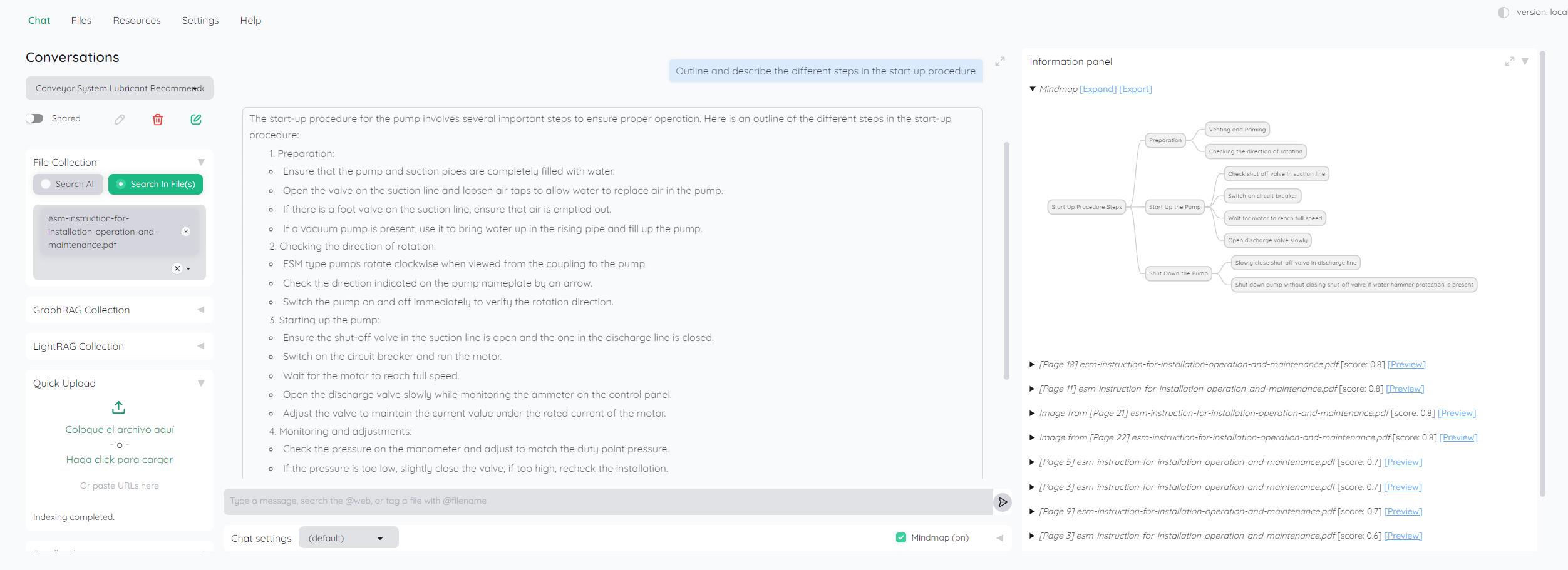
Machine-generated sensor data is another cornerstone of predictive maintenance, often complementing textual insights. NLP can bridge the gap between sensor data and human-readable reports by translating numerical patterns into comprehensible language. For example, automated alerts generated by IoT-enabled machines can be processed through NLP systems, which then contextualize these alerts with historical maintenance data and textual annotations from past events. This integration allows maintenance teams to make more informed decisions based on both quantitative and qualitative insights.
Additionally, NLP plays a pivotal role in extracting information from external sources such as academic research, industry standards, and online forums where engineers discuss maintenance challenges and solutions. By continuously analyzing this information, NLP systems can keep predictive maintenance models updated with the latest advancements and emerging failure trends.
As machine learning and NLP technologies continue to evolve, their integration with predictive maintenance in mechanical engineering will become even more sophisticated. The ability to process and understand textual data at scale enhances predictive accuracy, reduces unexpected failures, and extends equipment life cycles. Industries that adopt these technologies gain a competitive edge through improved operational efficiency and reduced maintenance costs. The synergy between NLP and predictive maintenance is transforming mechanical engineering by bridging the gap between human expertise and automated intelligence, creating a more resilient and data-driven approach to equipment management.