Undergoing development of an automatic defect detection system in manufactured parts, using convolutional neural networks to detect features in an image while simultaneously generating a high-quality segmentation mask for each instance.
Common defects include cavities, pores, lack of penetration, and other type of volumetric defects frequently found in castings and welded parts.
Detection of these defects can allow faulty products to be identified early in the manufacturing process, resulting in improvements in quality, as well as time and cost savings.
The defect detection system is trained and tested on publicly available X-ray dataset and is currently at an early development stage.
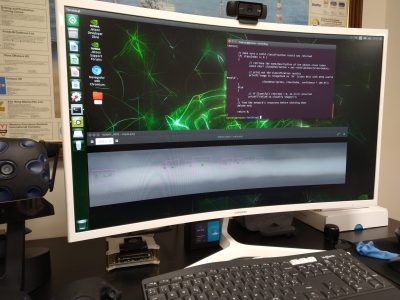
The classification method being used is binary (defect or no-defect) so the results being obtained are only useful for a first pass assessment of the quality of the weld. It would be possible, on a further iteration, to use a multi-class defect classification allowing much greater granularity and variety in the results (gas cavity, lack of penetration, porosity, slag inclusion, undercut, lack of fusion, etc).
